Generative Transformation
Mavengigs
Mavengigs is a global consulting firm providing consulting services for Mergers & Integrations (M&A) and Transformations. Through our network of independent resources and partners, we serve clients in USA and Europe. Mavengigs is a division of Panvisage Inc. (a holding company with interests in consulting, education, real estate and investments).
Generative Transformation
Generative AI is reshaping the approach to product development and utilization. This form of artificial intelligence, generative AI, holds the potential to be more transformative than any of its predecessors. Currently, it is already transforming content generation, and it holds the potential to reshape various aspects of business, including innovation, customer engagement, market analysis, employee training, market research, and software development.
Many business executives understand the significance of integrating Generative AI into their companies and customer-oriented applications. However some are uncertain about how to initiate this transformation and convert AI enthusiasm into tangible business benefits. Adopting a “wait and see” stance risks falling behind organizations actively embracing Generative AI. Using AI-driven efficiency improvements to reduce staff numbers is not the best use case. Generative AI Transformation should serve as a tool for redefining how business operates and reshape customer interactions.
A Large Language Model (LLM) (Generative AI) is a specific type of algorithm that utilizes deep learning techniques and vast datasets to comprehend, condense, and produce fresh content. Essentially, it takes in natural language input and forecasts subsequent words based on the context it has already processed, ultimately delivering a comprehensive intelligent response.
Gartner has predicted that by 2024, 40% of enterprise applications will have embedded conversational AI, up from less than 5% in 2020; By 2025, 30% of enterprises will have implemented an AI-augmented development and testing strategy, up from 5% in 2021; By 2026, generative design AI will automate 60% of the design effort for new websites and mobile apps; By 2026, over 100 million humans will engage robo colleagues to contribute to their work; By 2027, nearly 15% of new applications will be automatically generated by AI without a human in the loop. This is not happening at all today.
Generative AI is set to make a significant impact on a wide range of industries, including pharmaceuticals, manufacturing, media, architecture, interior design, engineering, automotive, aerospace, defence, medical, electronics, and energy. It will enhance fundamental operations by incorporating AI models. Furthermore, it will influence functions like marketing, design, corporate communications, training, and software engineering, enhancing the supporting processes that extend across various organizations.
As per Deloitte’s CEO survey, key focus will be on improving efficiency, automation of manual process, reducing operational cost, capture quality data, improve growth opportunities. In the realm of business, the distinctions between strategic, tactical, and operational decisions are increasingly becoming less clear, necessitating business leaders to continually reassess how they can utilize data and AI to enhance their decision-making processes.
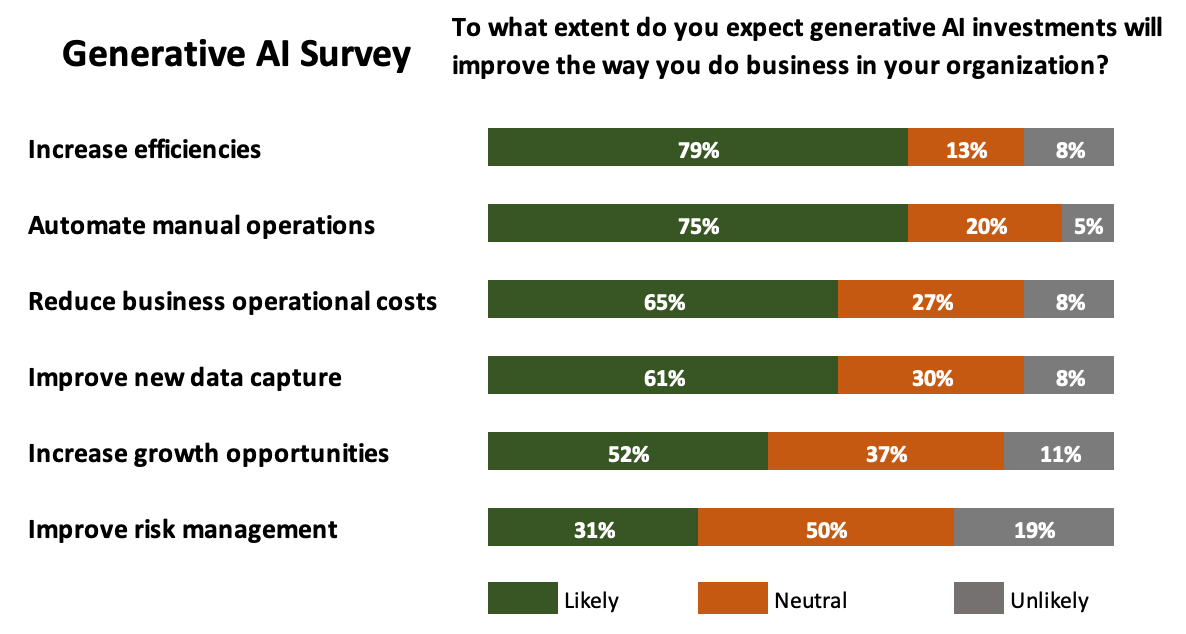
This is not about overhauling every decision; it’s about applying this restructured mindset to the most vital and influential decisions—those that cannot be efficiently addressed through traditional methods. The emergence of artificial intelligence (AI) and large language models (LLMs) will imminently influence the strategic planning process for businesses. As these technologies advance, they will alter the way leaders assess alternatives, predict results, and make crucial, long-term decisions.
Incorporating LLMs into this procedure can be a game-changing move. They have the capacity to offer insights and forecasts that contribute to more informed decision-making. They assist in creating decisions that are more interrelated, contextually relevant, and ongoing. Moreover, they contribute to optimizing the business at a broader level by engaging all relevant stakeholders
Decision Making
It’s vital to keep in mind that the core of decision-making is fundamentally a human endeavour. It revolves around understanding, empathy, and exercising judgment. It entails the evaluation of advantages and disadvantages, confronting uncertainties, and ultimately arriving at decisions that align with our values and goals. AI and LLMs serve as potent allies in this endeavour, but they don’t supplant the human element; rather, they collaborate and strengthen it. It is imperative to understand the category of decision making and its’ automation.
They can be categorized into 3 areas:
- Decision Support
- Decision Augmentation
- Decision Automation
Decision Support
Data and analytics serve as tools to assist human decision-makers by providing information, data, or visual representations. The ultimate decision is made by a human, drawing from a combination of principles and ethics, prior experience and potential biases, logical and reasoned thinking, emotional factors, and individual skills and decision-making style, whether it’s done independently, through delegation, or collaboratively. Machines, in this context, offer support to human decision-makers by providing visualizations, aiding in data exploration, delivering alerts, and various other forms of assistance.
Decision Augmentation
This type of decision-making involves offering recommendations to human decision-makers. This decision model encompasses both attended and unattended automation tasks, and there are several ways to augment decision-making. It can involve machines suggesting, and humans making the final decision. Alternatively, humans can propose, and machines execute the decision. In some cases, both human and machine collaborate in making the decision. Each of these scenarios has a distinct dynamic. Artificial intelligence is employed to generate recommendations and may also offer diagnostic analytics for human assessment and further exploration.
Decision Automation
Machines and devices can make independent decisions, but humans typically review these automated decisions after they have been executed to retain control over complex systems. Risks in this process are mitigated through predefined rules or through human decision authorities for exceptional or unforeseen cases. Autonomous decision-making by machines involves predictions, forecasts, simulations, rules, optimized algorithms, and other forms of artificial intelligence to drive the decision-making process.
The Future of Decision-Making with AI and LLMs
By incorporating AI & large language models (LLMs), one can extract valuable insights from unfolding events in real-time and make these insights accessible to subsequent models or business processes. LLMs are highly potent and versatile AI models. With the appropriate input prompts, integrating LLMs into production pipelines, enabling downstream systems to take real-time actions. One can also send real-time data directly to LLMs, enabling them to make decisions within the context of real-time information. More advanced workflows might even involve hosting multiple LLMs that act as both data producers and consumers in real-time scenarios.
Insights and Predictions
Strategic planning places a significant emphasis on the ability to anticipate future scenarios and offer valuable insights. Large language models (LLMs) are proficient in processing extensive datasets such as news articles, research documents, and company reports, enabling them to identify patterns and trends. This capability enhances the precision of forecasting market conditions, competitor strategies, and financial results when assessing strategic approaches.
Market Intelligence
Large language models (LLMs) have the capacity to analyze data, gaining insights into customer needs, identifying pain points, and comprehending competitor offerings. This market intelligence proves invaluable for strategy teams as they evaluate opportunities and potential threats, leading to a more comprehensive understanding of the landscape.
Product Development
Generative AI will empower enterprises to accelerate the development of novel products. These innovations may encompass swifter creation of new pharmaceuticals, the formulation of environmentally friendly household cleaning products, the invention of unique flavours and fragrances, the development of novel alloys, and the enhancement of speed and precision in medical diagnoses.
Quality Deliverables
Generative AI has the potential to enhance workers’ capabilities in creating and editing text, images, and various media forms. It can effectively summarize, simplify, and categorize content, generate, translate, and validate software code, and enhance the performance of chatbots. Currently, this technology excels in rapidly producing a diverse array of artifacts on a large scale.
Risk Mitigation
Generative AI’s ability to analyse and provide broader and deeper visibility of data, such as customer transactions and potentially faulty software code, enhances pattern recognition and the ability to identify potential risks to the enterprise more quickly.
Rapid Scenario Planning
Utilizing a Large Language Model (LLM), strategists can rapidly generate specific scenarios for in-depth exploration. This speeds up stress-testing strategies and contingencies, achieving results in a fraction of the time it traditionally takes.
Sustainability
Generative AI has the potential to assist enterprises in adhering to sustainability regulations, mitigating the risk associated with stranded assets, and integrating sustainability principles into decision-making, product design, and operational processes.
The Next Steps
Generative AI implementation will be rolled out in steps, with different speeds and priorities across organizations.
Typical steps would include the following
- Begin with decision intelligence in areas where essential business decisions demand enhancement, necessitating greater data-driven assistance or AI-powered augmentation.
- Alternatively, initiate the process where decisions can benefit from automation, allowing for scalability and expedited outcomes.
- Another approach is to focus on areas where current decision-making processes are most dissatisfactory, potentially leading to quick wins and improvements.
- Enhance decision-making by initially gaining a comprehensive understanding of the current decision-making processes and creating models to explore potential reengineering.
- Subsequently, integrate both human and/or AI decision-making capabilities into these processes, establishing a feedback loop to measure the outcomes and effectiveness of these improvements.
- Start Internally within the organization based on internal use cases.
- Perform POC and test extensively for an extended period.
- Establish protocols and guidelines to monitor biases and other trustworthiness issues. This involves validating outcomes and conducting ongoing tests to detect instances where the model deviates from its intended course.
- Address privacy and security.
- Ensure not to use sensitive data
Some use cases to start with AI & LLM
- Business content creation (text, image etc) and augmentation.
- Simplification and Professionalize text, Translation.
- Classification of Content like sentiments etc
- Chatbot performance improvement like conversation sentiment classification, flow generation
- Software Code generation

Creating a framework for Trustworthy AI often involves innovative problem-solving from corporate executives, rigorous examination at each step of the AI’s life cycle, and a dependable guarantee that the tools and the ecosystem supporting them adhere to the essential aspects of trust. Each company has its individuality and must confront hurdles and preferences linked to its business strategy and goals, leading to diverse chances for effectively harnessing AI capabilities. Therefore, there isn’t a universally applicable template for efficient AI procedures. Rather, it entails essential actions to design and enact processes for managing the company’s AI initiatives.
Strategies for managing AI-related risks and continuous assessments of model risks can aid the organization in readying itself for and shielding against external influences that might have detrimental impacts on both the model and the business strategy. Establishing a thorough and ongoing strategy for managing risks commences with the evaluation of potential risks. These evaluations then inform the development of a risk management plan, which forms the foundation for crafting controls and responses to mitigate those risks. This approach facilitates human oversight and decision-making. These resources and activities, when combined, play a vital role in bolstering the resilience and dependability of AI models and cloud infrastructure, addressing vulnerabilities in the system, and handling other critical facets of trust, such as privacy, safety, and security.
While these elements will probably make use of the current infrastructure, it’s crucial to integrate Trustworthy AI principles into their governance model as companies adjust to a business landscape where AI significantly influences fundamental business operations. By adopting this approach, organizations can pinpoint areas that need improvement and devise viable solutions while simultaneously advancing the program, enhancing its pace, and boosting efficiency.
- Data governance and processing: The overall governance model of data used for AI modelling which includes data acquisition, data storage, data quality, data labelling & annotation, data privacy & compliance, data security, policies & responsibilities.
- Security: Securing data used by AI models (as well as the models themselves) from adversarial attack.
- Governance Process: Define Ops (AL/ML Ops, LLMOps, DevOps) model from ideation and deployment and control.
- Model development and evaluation: Evaluating & validating AI model performance, monitoring risks & processes, and controls on an ongoing basis.
- Controls to ensure the AI use cases do not exhibit biases that could be illegal, discriminatory, or unethical.
- Decision Automation: How much authority or judgment is delegated to the relevant AI model.
AI and LLMs offer significant potential, but they also come with drawbacks such as potential job displacement and ethical considerations. Their adoption demands strategic planning and effective change management. It’s crucial to bear in mind that LLMs are tools designed to aid decision-making processes rather than replace human judgment and expertise. Therefore, their utilization should be coupled with human oversight and domain-specific knowledge to ensure responsible and effective decision-making.
Please contact us today!
Mavengigs
16192 Coastal Highway, Lewes, DE 19958
Contact Us
Ph: (310) 694-4750, sales@mavengigs.com